These Folks Get It
Learn why more than 85,000 customers build better software, faster, with Sentry.
Try Sentry For Free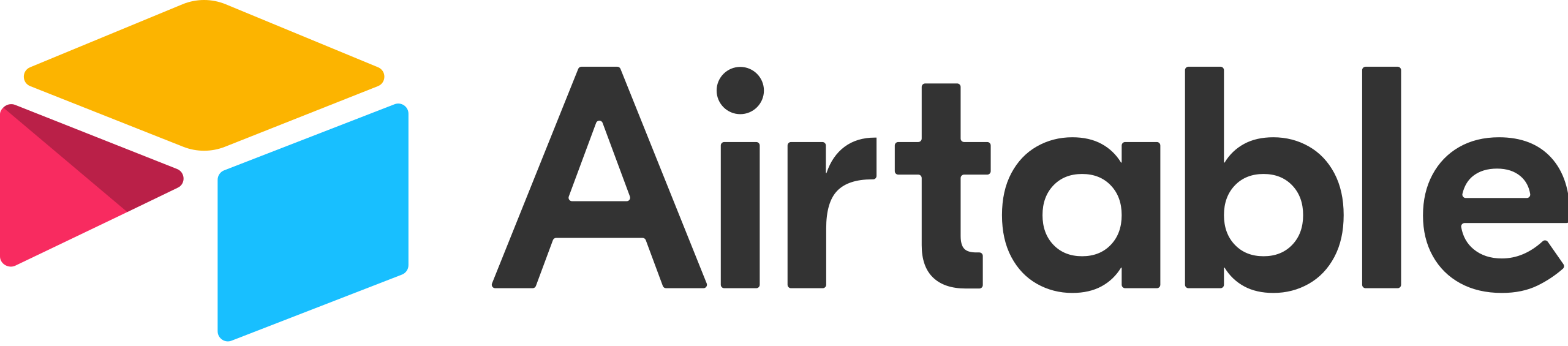
“In the past, this type of investigation would require pivoting between tools to get alerted and it would take 10-15 mins to correlate it with impacted customers. Now, we can do the same thing in Sentry in a couple of minutes.”
Doug ForsterSoftware Engineer
Read On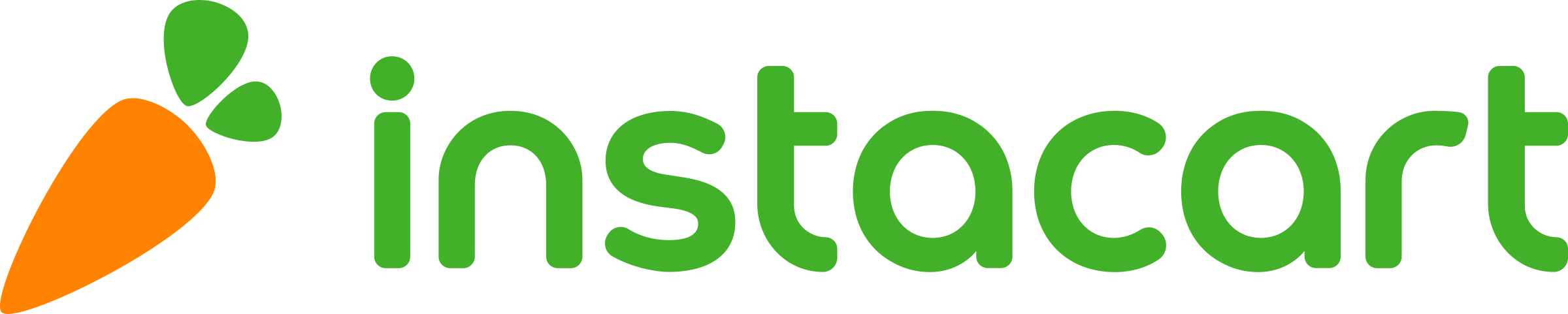
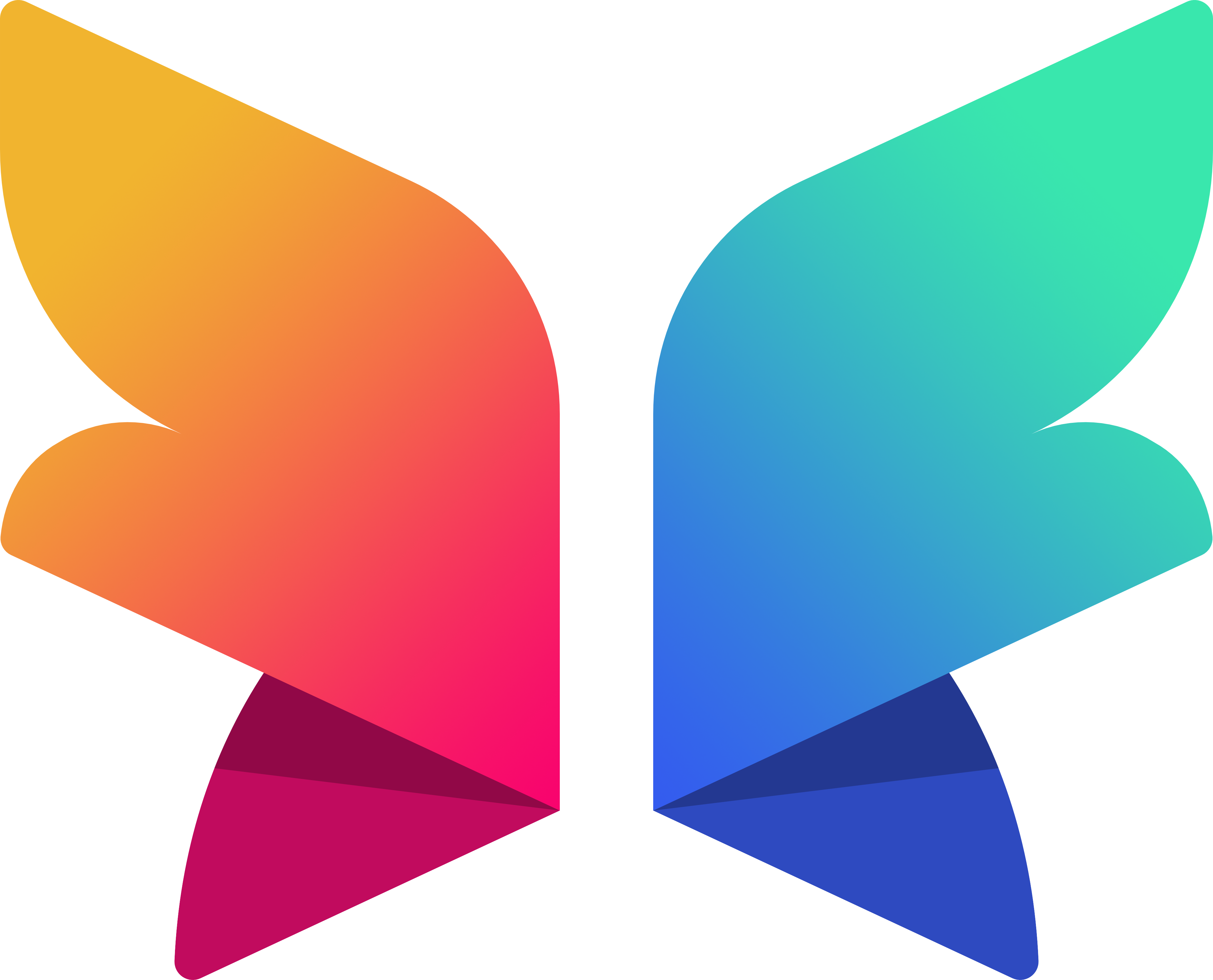
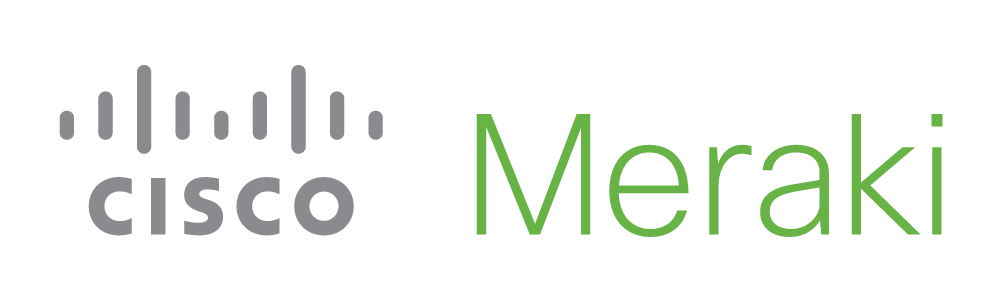
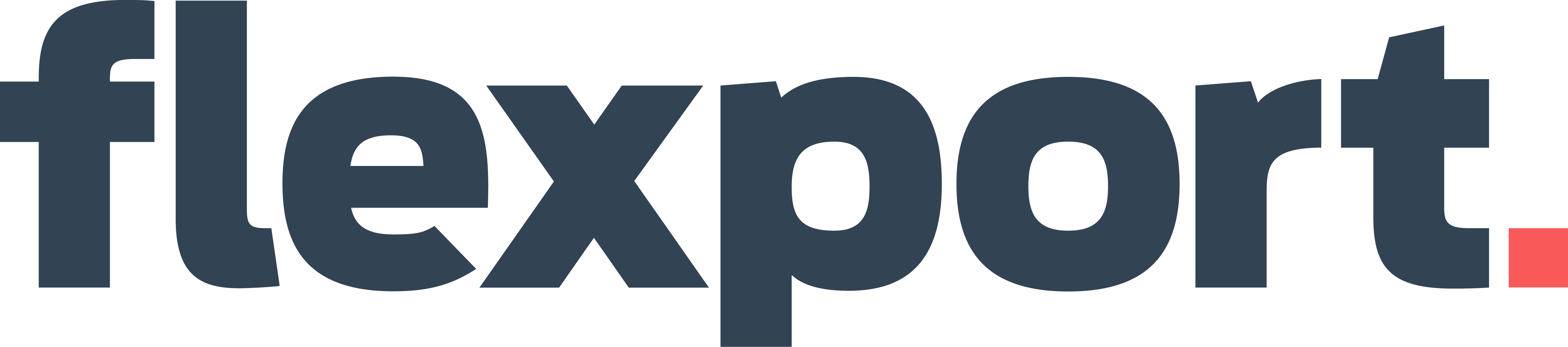

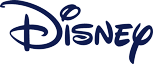

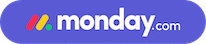
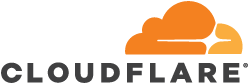


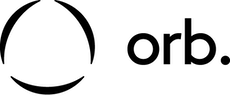
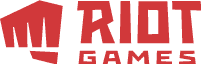
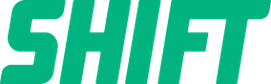
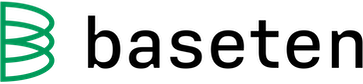


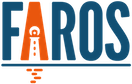
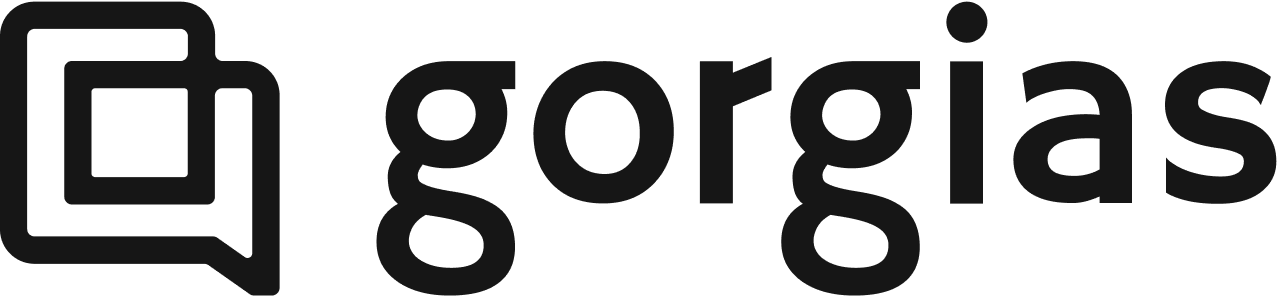
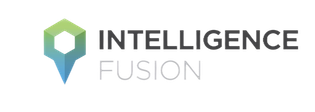
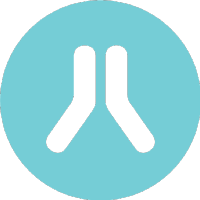
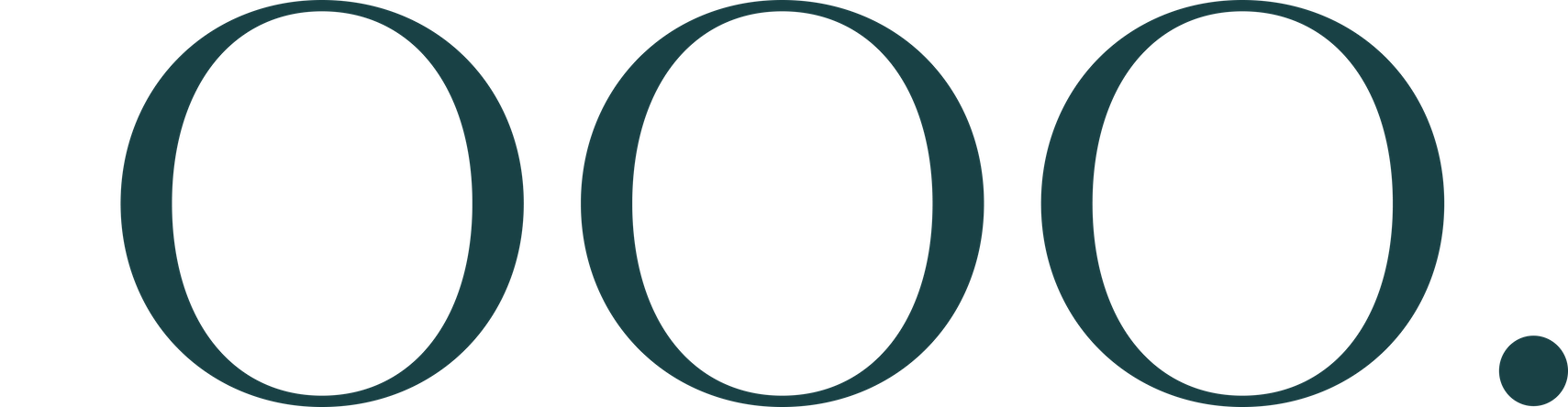
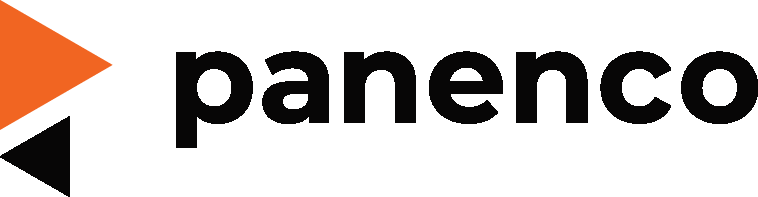
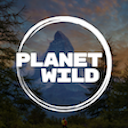